Zhun Zhong
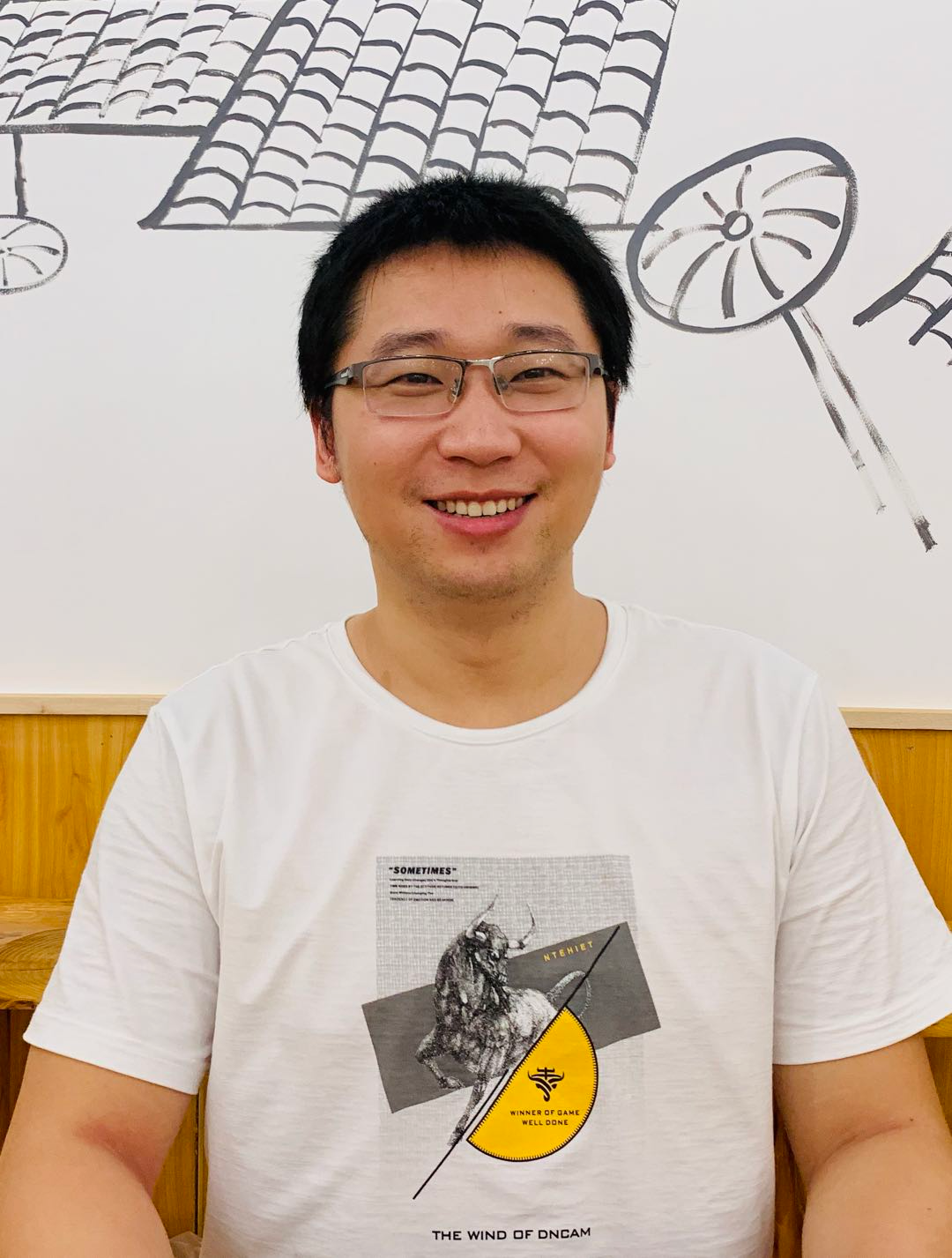
--------------------------------------------------------------------------------------------------------
Assistant Professor
School of Computer Science
University of Nottingham
--------------------------------------------------------------------------------------------------------
About Me
Experience: I am currently an Assistant Professor at the University of Nottingham (UoN). I was an Assistant Professor (2022-2023) and a Postdoc at University of Trento (UNITN, 2020-2022) working with Prof. Nicu Sebe. I received my Ph.D. degree in 2019 from the Cognitive Science Department at Xiamen University under the supervision of Prof. Shaozi Li. I was also a joint Ph.D. student in 2017-2019 at University of Technology Sydney under the co-supervision of Prof. Yi Yang and Dr. Liang Zheng. I received the M.S. Degree in Computer Science and Technology in 2015 from China University Of Petroleum, Qingdao, China. I received the B.S. degree from the Information Engineering Department, from East China University of Technology in 2012.
Research Interests: Our Lab for Intelligence and VisiON (LION) commits to designing trustworthy intelligent visual recognition systems for real-world applications. To achieve this goal, we mainly focus on the areas of data augmentation, unsupervised/semi- supervised learning, federated learning, domain generalization, domain adaptation and novel class discovery, and investigate their advantages in visual tasks, such as object retrieval, image classification, semantic segmentation, etc.
Join Us: I am looking for highly-motivated Ph.D. students starting from September 2024; I also welcome visiting students/research assistant.
I am also a guest researcher at UNITN (MHUG led by Prof. Nicu Sebe), in which we are hiring highly-motivated Postdoc/Ph.D. students/visiting students/research assistant.
Drop me an email if you are interested in the above positions. Please use the subject format [Name_Position_AppliedUniversity]. For example, [Jack_PhD_UoN], [Mike_Postdoc_UNITN]. [English Version] [Chinese Version]
News
May 30, 2024 | I am invited as AE for Computer Vision and Image Understanding。 |
---|---|
May 1, 2024 | One paper is accepted by ICML 2024. Congrats to Chentao. |
Apr 15, 2024 | I am invited to serve as an Area Chair (AC) for NeurIPS 2024. |
Mar 25, 2024 | Four papers are accepted by CVPR 2024. Congrats to all. |
Feb 1, 2024 | I am invited to serve as an Area Chair (AC) for ICML 2024. |
Jan 23, 2024 | One paper about Vocabulary-free Fine-grained Visual Recognition is accepted to ICLR 2024. Congrats to Mingxuan. |
Oct 30, 2023 | I am invited to serve as an Area Chair (AC) for ECCV 2024. |